Auditability through explainable AI
Feb 3, 2025
As AI systems become increasingly embedded in business-critical decision-making, one fundamental challenge persists: explainability. How do organisations trust and validate AI-driven answers when the reasoning behind them is opaque? At UnlikelyAI, we believe that robust decision-making requires more than just high accuracy—it requires auditability. Our product is designed to provide transparent, accountable, and reasoned outputs, ensuring AI-driven decisions can be scrutinised both internally and externally.
Beyond Yes and No: The Power of "Don't Know"
Unlike many AI solutions that provide binary answers, UnlikelyAI classifies responses into three categories: Yes, No, and Don't Know. This structured approach acknowledges the uncertainty in complex decision-making, preventing overconfidence in AI-generated results. But more importantly, every answer is accompanied by an explanation, outlining the reasoning behind the response.
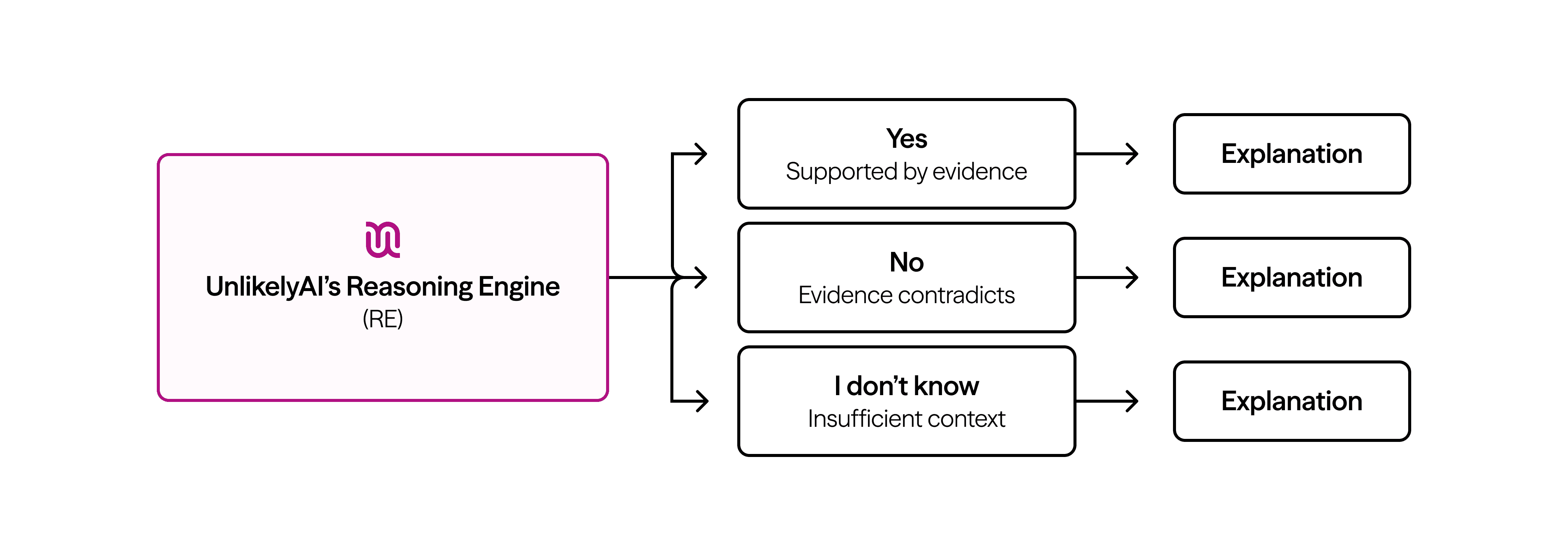
This transparency is critical for regulated industries such as banking, insurance, and healthcare, where compliance demands not just decisions, but justifications. Our approach contrasts sharply with traditional Large Language Models (LLMs), which often generate answers that sound plausible but lack verifiable reasoning (Zhuo et al., 2023).
The Risk of Opaque AI in Business-Critical Decisions
A lack of explainability isn’t just an academic concern—it has real-world consequences. Research indicates that AI errors in financial decision-making could lead to misallocated loans and credit denials, with potential economic losses in the billions. Similarly, in healthcare, a misdiagnosis due to AI hallucinations could significantly impact patient outcomes.
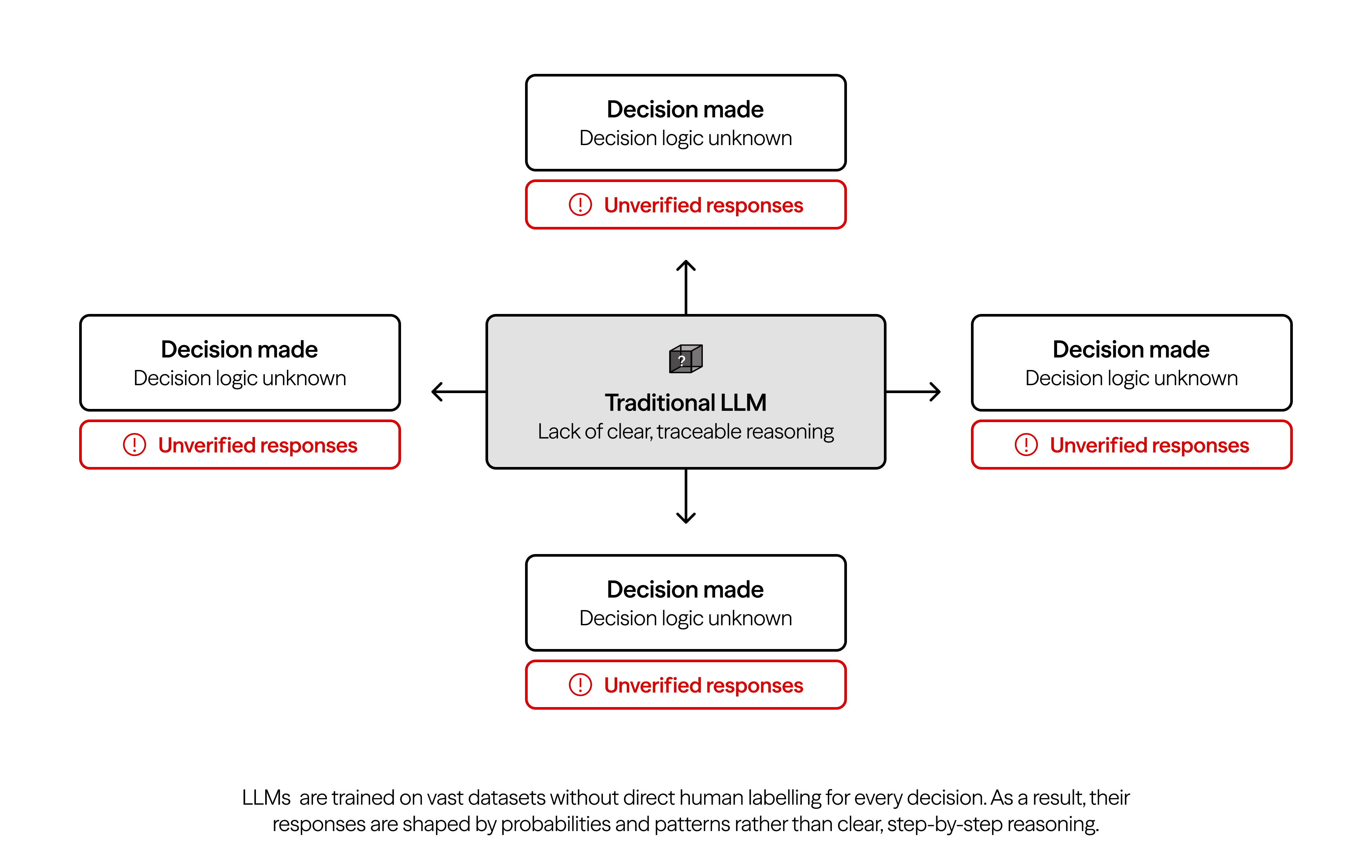
A 2023 survey by McKinsey found that 67% of executives worry about AI’s ability to provide accountable decision-making (McKinsey, 2023), yet only 20% of AI implementations include mechanisms for explainability. Without clear reasoning, organizations are exposed to both regulatory risk and reputational damage.
Auditability: The Missing Link in AI Decision-Making
UnlikelyAI’s platform directly addresses these risks by creating an auditability log — a detailed record of every decision made, including:
Supporting evidence extracted from trusted sources
Reasoning steps outlining the logic behind the conclusion

This audit log can be reviewed by internal compliance teams, external auditors, and regulatory bodies, ensuring transparency at every level.
How UnlikelyAI Differs from Standard LLMs
Traditional LLMs, including GPT-based models, struggle with explainability. Studies have shown that even well-tuned LLMs can generate fabricated citations up to 20% of the time (Nie et al., 2024). While Retrieval-Augmented Generation (RAG) approaches improve factual grounding, they still lack structured reasoning and auditability logs that are crucial for regulatory compliance.
Recent advancements, such as OpenAI’s o1 model, have improved reasoning capabilities in AI. However, despite these improvements, challenges persist in regulated industries. The lack of transparency in decision-making remains a critical issue, as compliance and accountability require clear justifications for AI-driven actions. Moreover, research highlights concerns over misleading or deceptive outputs, reinforcing the necessity for robust auditability mechanisms in business-critical applications. UnlikelyAI mitigates these risks by ensuring that every response is backed by traceable logic and verifiable evidence.