The AI solution that gives complete control
Feb 5, 2025
Integrating LLMs into your business workflow introduces inherent risks—hallucinations, non-deterministic results, and a lack of auditability. These issues are exacerbated by the fact that traditional LLMs provide little to no direct control over their responses.
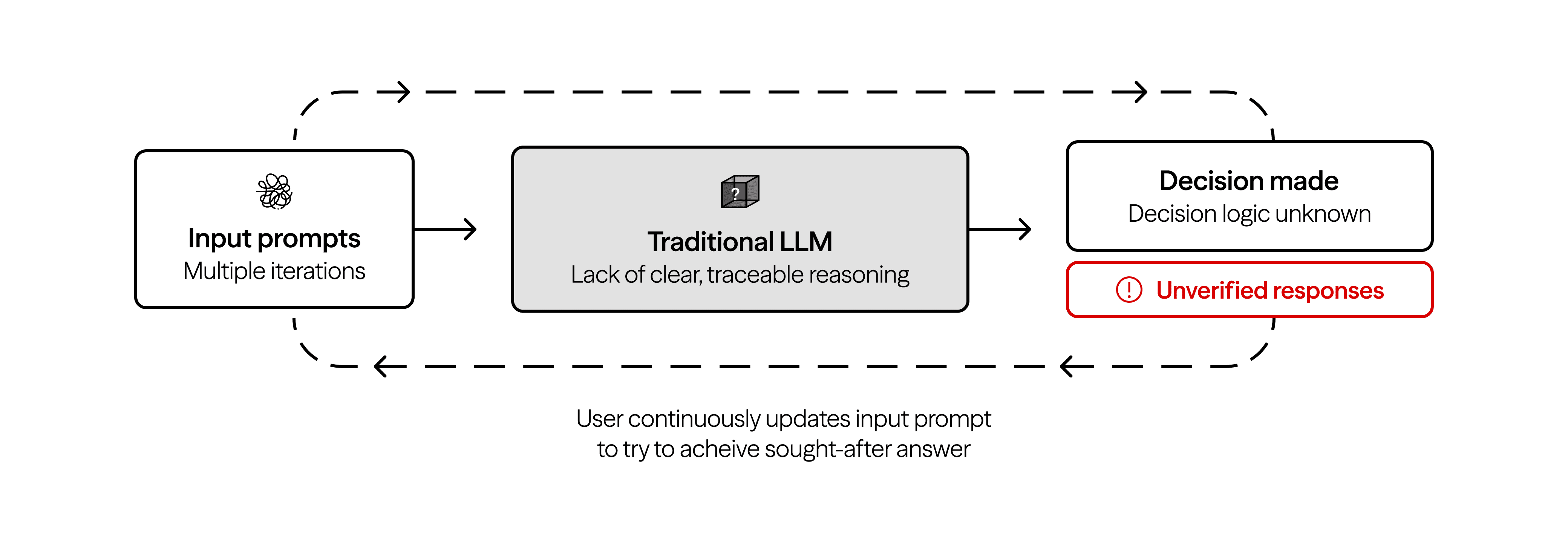
This lack of control has given rise to the concept of prompt engineering, where users craft ever more complex prompts in an attempt to create a small pocket of reliability within the vast, unpredictable landscape of an LLM’s knowledge and biases. The most you can do is nudge a model in the direction you want - you can never be sure of its output.
A Better Approach: Context, Control, and Interrogation
At Unlikely AI, we take a fundamentally different approach—one that prioritizes your control over AI behavior. Rather than relying on ad-hoc prompt manipulation, we provide a structured way to define and refine AI understanding, ensuring predictability, auditability, and transparency at every step.
Rather than us training our system on data with a broad, unspecific selection of data, you teach the system exactly what you want it to know.
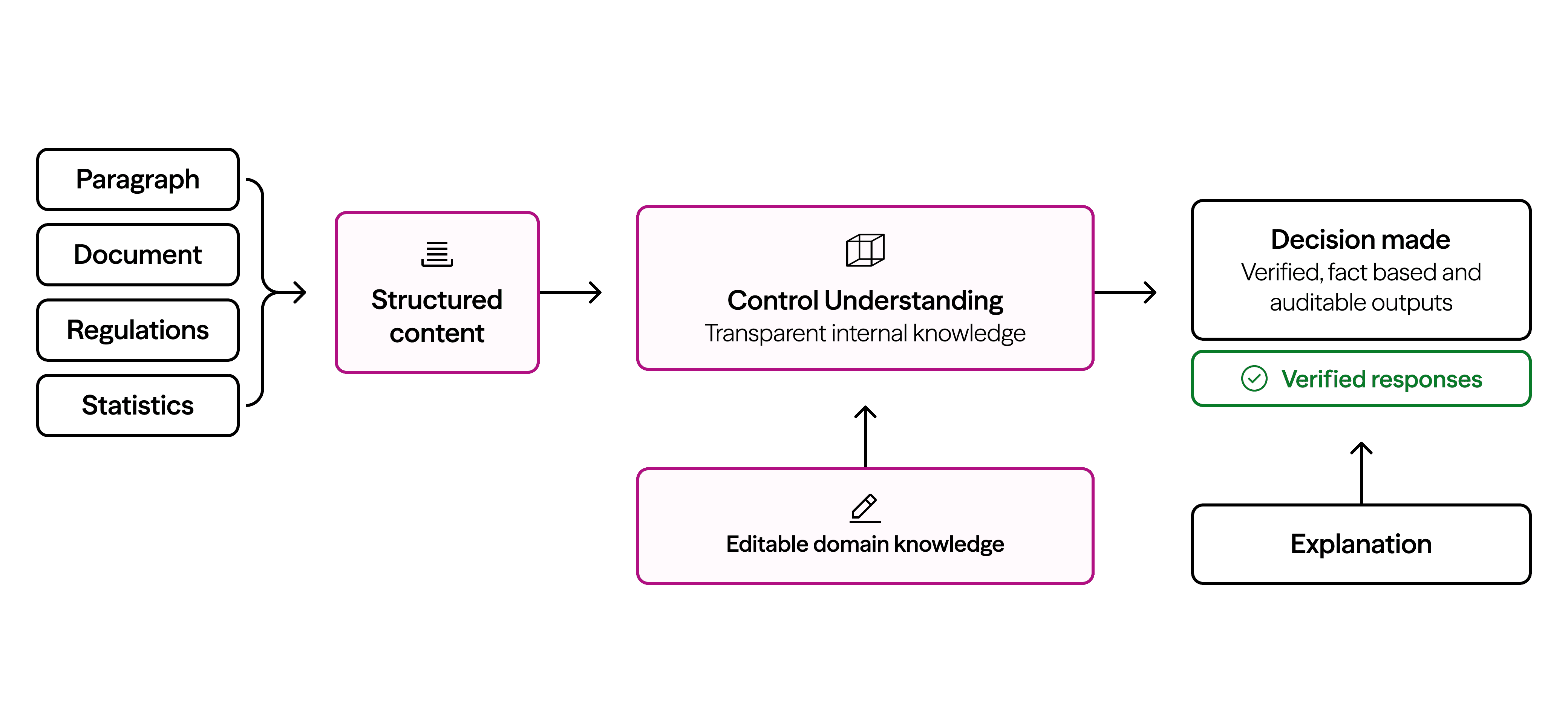
Provide context
The first step in gaining control is ensuring that the AI understands what matters to you. By teaching our system, you can provide the system with context in any format—documents, structured data, or natural language instructions. The AI then translates this context into a structured domain representation that you can review, test, and modify before any reasoning takes place. This removes any guesswork and ensures transparency in how the AI processes information. With the ability to update domain knowledge as needed, the system remains flexible and adaptable to changing business needs.
Control understanding
Once your domain understanding is established, you can see exactly what the AI has learned—in plain English. If something isn’t quite right, simply make a quick edit. Need to expand the AI’s knowledge? Easily add additional context to refine its scope and accuracy. This provides full visibility into the AI’s internal knowledge, allowing for rapid adjustments with minimal effort, ensuring increased precision and alignment with business requirements.
Interrogate responses
Once you have a working domain understanding, you can start asking the AI questions and evaluating its responses. Each answer includes:
Answer: the outcome that the Reasoning Engine has reached based on the domain understanding and the information provided in the query.
Explanation: A step-by-step reasoning process that outlines why the system arrived at the given answer.
Reasoning graph: A visual breakdown of how each piece of information was used to reach the conclusion, ensuring complete transparency and auditability.
By eliminating the black-box nature of traditional AI, every answer is backed by a clear, logical explanation. This ensures auditable reasoning, allowing users to track and verify decisions while continuously improving the AI’s accuracy over time.
Why This Matters
Unlike traditional LLMs that provide unpredictable and opaque results, Unlikely AI’s neurosymbolic approach ensures you remain in control. By defining the context, verifying understanding, and interrogating responses, you gain an AI system that is predictable, reliable, and fully auditable.